Data Analytics Shines Light on Ceramic Processing
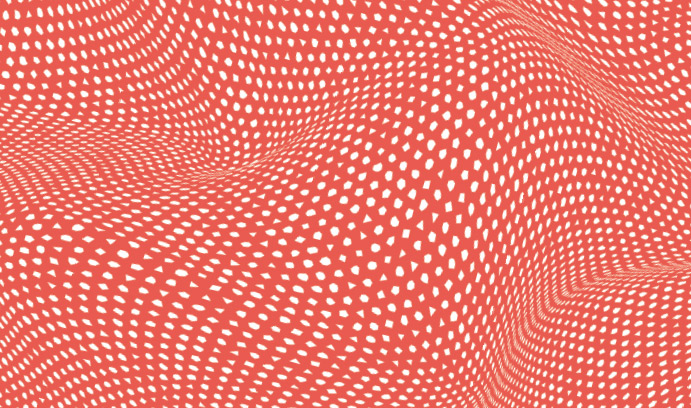
The sintering of ceramics, says Jeffrey M. Rickman, can be likened to the baking of desserts.
The successful preparation of a pie or cake depends on a combination of factors, including the time and temperature of baking, the ingredients used, and the order and manner in which the ingredients are mixed together.
So it is with the heating and pressure that transform ceramic powders into a wide array of solid products. To make a part, such as an artificial joint, with the requisite properties—strength, ductility, transparency, durability, conductivity, etc.—one must correctly calibrate a multitude of input variables, or ingredients, says Rickman.
“A ceramic powder can have different additives and chemistries,” says Rickman, a professor of materials science and engineering and also of physics. “The powder can be processed in different ways, at different temperatures, for different lengths of time and under different pressures.
“These are the ingredients that will determine what the fully baked result will be. If you vary the ingredients, you can vary the final result. And with ceramic materials, there’s a very high sensitivity of the output properties to the input variables.”
Rickman and his research group have developed a versatile, data-analytic approach that more accurately predicts the relationships between inputs and outputs for problems in which those relationships are both linear and nonlinear.
Their approach, a combination of canonical correlation analysis and Monte Carlo simulation (CCAMC), identifies the combination of input variables that most closely correlates with desired outputs. The group has applied CCAMC specifically to materials processing problems but the approach is general and can be applied to other fields as well.
The group has validated CCAMC with two problems involving technological applications. First, CCAMC has determined the relationship between various ways of processing doped polycrystalline aluminas and the resulting microstructural properties of the aluminas. Second, CCAMC has successfully related microstructural characteristics to electrical and optoelectronic properties in thin-film solar cells that are based on copper-indium-selenium absorbers.
CCAMC, Rickman says, promises to give ceramic manufacturers greater control over a multitude of input variables and the relationships among these variables. This will enable engineers to make ceramics that more reliably meet the specifications of customers and to detect and correct processing problems more quickly.
Rickman and his group reported their results recently in the journal Nature Computational Materials in an article titled “Data analytics using canonical correlation analysis and Monte Carlo simulation.”
In addition to Rickman, the authors of the paper include Yan Wang, who completed his Ph.D. in materials science and engineering at Lehigh this year and is now a research scientist at Corning Inc.; Martin P. Harmer, professor of materials science and engineering at Lehigh; Anthony D. Rollett, professor of materials science and engineering at Carnegie Mellon University; and Charles Compson of Almatis Inc., an alumina manufacturer in Leetsdale, Pennsylvania.
“Our approach is easily implemented and provides in many cases, with relatively little computational cost, combinations of variables that are strongly correlated,” the group wrote in Nature Computational Materials.
The Monte Carlo Edge
Canonical correlation analysis (CCA) is well established in the social sciences and other fields, says Rickman, but has not yet been widely used in the natural and physical sciences. CCA allows researchers to take problems with large numbers of inputs and outputs and to identify the relatively few variables that are significant.
“CCA asks what combination of inputs is most related to the combination of outputs that you want,” says Rickman. “It lets you reduce large numbers of inputs and outputs to only those that are really important. Let’s say you have a problem with 10 inputs and 20 outputs. After you analyze it, however, you may find only three that are really significant, that are most correlated to input-output and need to be examined closely.”
A drawback with CCA is that it detects only linear relationships. This presents a challenge with ceramic processing and other problems with multiple inputs and outputs whose relationships are often nonlinear and thus more mathematically complicated.
“CCA finds linear combinations of inputs related to linear combinations of outputs,” says Rickman. “If you have, say, three inputs and three outputs, CCA will find the linear combination of inputs that is most related to a linear combination of outputs. This is useful, but it doesn’t take into account more complicated types of relationships.”
To overcome this drawback, Rickman’s group employed Monte Carlo simulation, a mathematical technique that accounts for risk in quantitative analysis and in decision making. Monte Carlo simulation is used in finance, energy, insurance, transportation and other fields.
“We have modified CCA by adding Monte Carlo simulation to it,” says Rickman. “Monte Carlo allows us to test many different nonlinear relationships in a systematic, sequential way. It tells us if a specific relationship is more strongly correlated than was previously thought.
“For example, you might think a specific output is dependent on an input variable x. It might not be x, however, it might be x squared or x cubed or x to the fourth. Monte Carlo lets you vary that exponent systematically, over and over, to find the exponent that works best.”
Accounting for Abnormal Grain Growth
Much of the complexity involved in the processing of ceramics, says Rickman, is caused by the abnormal growth of the grains, or crystallites, in polycrystalline ceramic materials. Under a microscope, the microstructure formed by these grains appears like a mosaic of tiles. The size and distribution of these grains are critical—they determine the properties of a ceramic and whether it meets the specifications desired by the end user.
As ceramic powders are sintered, says Rickman, some of these grains can become unusually large relative to the sizes of neighboring grains. This abnormal grain growth influences the bulk properties of the ceramic, usually in a negative way. Rickman’s group published an article on the topic in 2016 in the journal Acta Materialia. Titled “Parsing abnormal grain growth,” it was written by Rickman, Harmer, Rollett and Abigail Lawrence, the lead author, who earned her Ph.D. from Lehigh in 2016 and is now a senior scientist with Medtronics in Minneapolis.
Abnormal grain growth can be caused by additives which are introduced, often in minute amounts, into the powder and which have different chemistries. Other causes include the duration, temperature and pressure of sintering.
“We need to understand why and how abnormal grain growth comes about,” says Rickman. “But in systems with all kinds of complicated additives, we can sometimes gain only a primitive understanding. If you have 10 additives, they could be in different proportions, have different chemistries and sit in different locations. Add in time, temperature and pressure and you can get all kinds of different inputs and different outputs.”
Abnormal grain growth, says Rickman, can be costly to a ceramics company by causing a change in properties.
“A company might make multiple batches that meet customers’ specifications. Then, during the processing of one batch, everything might go haywire and nobody knows why. The same procedure and recipe were followed, but maybe a tiny bit of foreign material got into the powder. Maybe there was a small change in the procedure that had huge effects.
“That’s the real bane of the ceramics profession. A mistake can cost you a lot of money because you’re making a product that isn’t useful to customers. So you have to have some understanding, at a very high level, of what happens during the processing of ceramic powders.”
In dealing with processing challenges, says Rickman, ceramic manufacturers have traditionally relied on the expertise borne of experience. CCAMC goes further by leading to a “substantial enhancement of correlations” between inputs and outputs.
“CCAMC lets you test more complicated possibilities, not just the one or two things that you have some intuition about, but five or 10 factors, some of which you have intuition about, some of which you don’t. All of these can all act together in some complicated way that you wouldn’t guess.”
The group is currently identifying other problems that will benefit from CCAMC.
The CCAMC project is related to Lehigh’s new Nano/Human Interface Presidential Engineering Research Initiative, a multidisciplinary endeavor that seeks to improve the ability of scientists to visualize and interpret the vast amounts of data generated by scientific research.
Story by Kurt Pfitzer
Illustration by Karan Singh
Posted on: