Hybrid Approach Optimizes Grid
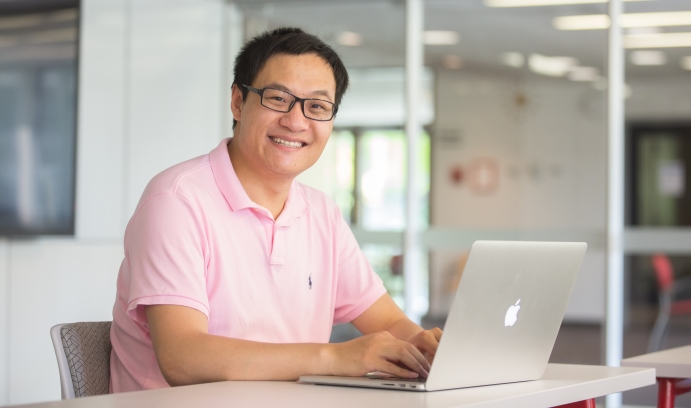
Jie Liu, a Ph.D. candidate in industrial engineering, recently received IBM’s Ph.D. Fellowship Award, one of the industry’s most competitive sources of funding for Ph.D. students. (Photo by Christa Neu)
This is especially true, says Liu, a Ph.D. candidate in industrial engineering, for the power grid, which directs electricity from power stations to consumers. Electric power should flow through the grid in a way that utilizes as efficiently as possible the resources that are used to produce the power.
This streamlined flow, says Liu, is made possible by machines that process large quantities of data in real time and make optimal decisions.
Liu and his colleagues, Martin Takáč, assistant professor of industrial and systems engineering and Liu’s Ph.D. adviser, and Jakub Mareček of IBM Research, have made considerable progress in solving optimal power flow problems in the past two years.
For his contributions, Liu recently received IBM’s Ph.D. Fellowship Award, one of the industry’s most competitive sources of funding for Ph.D. students.
A power system, say the researchers, often contains many power stations, which produce electric power, and a central entity, called the transmission system operator, which coordinates the production and transmission of power.
The goal of the operator is to transmit electricity from power stations to customers with maximum efficiency and minimal losses. If a wire carries too much electric current, it incurs losses and could overheat, possibly causing a blackout.
Operators of power plants and transmission systems cannot choose where power will flow, say the researchers, because power follows the laws of physics. But they can decide where to generate power and how to set the transformers along the way.
The physics of the alternating-current model of power flows makes these decisions difficult to make, says the group. But they correspond to polynomial optimization problems (POPs), which are a hot topic today in the field of mathematical optimization.
Until recently, researchers could apply the so-called Newton method to POPs to obtain a solution quickly. Or, as suggested by Mareček and his colleagues, they could solve a sequence of surrogate problems to obtain the best possible solution.
The second approach often took too long, though, causing Liu, Mareček, and Takáč to seek conditions under which one could switch from the surrogate problems to the Newton method without obtaining solutions that would be wrong. Using recent developments in numerical algebraic geometry, the group has developed such conditions and designed methods to test them efficiently. One can therefore solve the surrogate problems quickly and, when it is safe, switch to the Newton method.
“This revolutionizes the field of polynomial optimization,” says Mareček.
The first in a series of papers by the group, “Hybrid Methods in Solving Alternating-Current Optimal Power Flows,” has just been accepted in IEEE Transactions on Smart Grid. That article is coauthored with Alan Liddell of the University of Notre Dame. It can also be viewed here.
Liu enrolled at Lehigh in 2013 after completing his M.S. in mathematics from the State University of New York at Buffalo. He holds a B.S. in mathematics from Nankai University in Tianjin, China.
Prior to receiving the IBM Ph.D. Fellowship, Liu received the Dean’s Doctoral Assistantship and Dean’s Fellowship from Lehigh’s P.C. Rossin College of Engineering and Applied Science, the Gotshall Fellowship from Lehigh, and the American Express Machine Learning Contest Award.
At Lehigh, Liu is part of a research group called Optimization and Machine Learning (OptML), which includes Takáč; Katya Scheinberg, the Harvey E. Wagner Endowed Chair Professor of Industrial and Systems Engineering; and Frank Curtis, associate professor of industrial and systems engineering.
Students in the OptML group receive support to present their work at international conferences and are encouraged to do industrial internships. Liu has worked with Siemens Corporate Research, Mitsubishi’s Electricity Research Laboratories (MERL), and Argonnes National Laboratory near Chicago, outside of IBM.
“These are amazing opportunities,” he says. “These are different companies, totally different. These internships connect us to industry. They give us the chance to do something we’re interested in and to learn new knowledge at the same time.
“The professors in our group are very supportive. They really help us learn how we can contribute and make an impact.”
Story by Kurt Pfitzer
Posted on: